insight
Kubrick consultant Brian Fang received a comprehensive look into the latest on all-things generative AI at the Applied Intelligence Live Conference in Austin, Texas. Fang, who currently supports one of Kubrick’s global energy clients, provides us with a breakdown of key takeaways from BENlabs Chief Technology and AI Officer, Tyler Folkman, that individuals and businesses can apply to leverage generative AI’s applications.
Cutting-edge technologies, like generative artificial intelligence (generative AI), have ushered in a new era of innovation, reshaping how we work and create, and redefining the limits of what is possible. Generative AI, put simply, can be defined as systems designed to learn patterns and structures from vast datasets, and then use that knowledge to recreate those structures in a compelling and convincing manner. During a talk at the Applied Intelligence Live Conference titled “Generative AI: The Game Changer in Entertainment and the Roadmap to Staying Ahead,” Tyler Folkman, the Chief Technology and AI Officer of BENlabs (formerly BENGroup), delved into the best practices for incorporating generative AI on a wider industry scale and for everyday usage.
To start things off, Folkman shared valuable insights into the strategies employed by BENlabs, which leverages data and AI to understand human attention and behavior in the context of marketing in the social media space (though these approaches have applications much wider than just digital marketing). These strategies were broken down into four main points: (1) contextualizing your data, (2) prioritizing data integrity, (3) compiling your AI toolkit, and (4) looking for predictability to accelerate AI adoption.
Contextualize Your Data: To harness AI effectively, it's crucial to contextualize your data. This allows businesses to ascertain true value from their datasets with insights into certain behaviors; namely, into consumer behavior. BENlabs have revolutionized consumer behavior analysis with the concept of “social neighborhoods” that people live in, connecting to one’s media preferences. For BENlabs, contextualizing data with social neighborhoods in mind will ensure that their marketing lands with the right audiences; however, businesses across industries can benefit from this strategy. Kubrick Consultant Maciej Dabrowny, a Data Scientist for a global banking institution, contextualizes data to predict customer behavior that will maximize resources and predict customer churn. By developing propensity models from modern data capabilities, “the impact is that you’re not throwing money away at a problem and hoping it solves it. You know how many people you need to employ,” explained Dabrowny, “or how much you need to spend training people, so [demand] is fully realized and you’re not wasting any resources.”
Prioritize Data Integrity: Data quality is of paramount importance. Folkman, who holds a Master’s Degree in Computer Science from UT Austin, emphasized the need to ensure that the data being analyzed and processed is ‘clean,’ representing real people (as opposed to bots). Additionally, filtering out bad data is essential to avoiding wrong insights. The potential for AI to combine human-like learning with machine-like scalability was revealed, highlighting AI’s promise in both predictability and analytical prowess. By ensuring their data is clean and accurate, organizations can confidently scale data capabilities.
Compile Your AI Toolkit: Rather than attempting to reinvent the wheel, Folkman encouraged businesses to build upon existing AI systems, creating comprehensive toolkits that accelerated AI adoption. If businesses shift their focus towards compiling AI toolsets and datasets that have already been going through stages of improvement and implementation, they may position themselves well to stay ahead. So, by building upon these existing AI systems and technologies, organizations are better able to accelerate their AI adoption and development processes.
Look for Predictability: It is essential to have a clearly defined purpose for improving AI (e.g. improving a company’s bottom line). AI's unique strength lies in its prediction capabilities. While humans can make predictions, we are limited by the volume of data we can process. AI, on the other hand, excels at predictability, because it can process large amounts of data at rapid rates. Businesses should aim to build AI systems that provide predictive power in addition to analytical capabilities of historical data.
AI’s Potential Perils, Promises, and How to Stay Ahead
Shifting gears slightly, Folkman went on to explore both the potential perils and promises of generative AI.
The first potential peril that Folkman stressed was the idea of ‘hallucinations,’ emphasizing the risk of relying entirely on AI-generated information without verification. In most cases, generative AI will not simply tell you, “I don’t have that information.” Instead, it will ‘hallucinate’ generating a confident response to a prompt, which may sound factual, even if there was no underlying data to support its own answer. One such example shared by Folkman is that of a lawyer who used an AI tool for legal research and inadvertently cited fictitious precedent to the judge. Generative AI tools like Chat GPT can be a great way to discover new concepts in an approachable way, with metaphors and well-explained breakdowns that serve as an easy introduction. However, because of the potential for hallucinations, this information always needs to be cross-checked with verified or reputable sources.
The potential for misinformation, such as deepfakes, was also addressed as a peril with severe harm and consequences; Folkman invited the audience to imagine the risk of deepfake videos being used as evidence in legal cases, for example. Furthermore, Folkman pointed out perils regarding non-differentiated content and IP infringement. Using the same AI as everyone else can result in content that lacks uniqueness, making it feel generic and unremarkable to consumers. And, ongoing legal disputes regarding the use of data to train generative AI models (without proper consent from businesses and individuals) were also stressed.
It is important to make sure that the risk of these potential perils can be reduced as much as possible by way of putting guardrails in place. In their most literal sense, guardrails exist to keep you from falling off the track. But, on the other hand, they are just guardrails: still providing the freedom and space to experiment, make mistakes, and explore – but preventing you from veering off track. In the paper, “How should Data Management adapt to ensure compliant & ethical Artificial Intelligence?,” Kubrick Data Management consultants discuss the need to have these guardrails, or guidelines, to combat biases, protect data ownership, for regulatory compliance, and the interdependency across these issues. “It is important, when developing or using AI, to recognize the associated ethical and compliance-based issues it may cause,” the consultants noted. “These issues are multi-faceted, concerning numerous groups within an organization, including the compliance and risk team, the legal team, and the data team. Thus, AI Data Management must involve technical teams, users, and business leaders in the DM process to ensure responsible ethical practices and not become siloed to be governed by a data team.” With the necessary guardrails in place, businesses will be able to explore data capabilities in a safe and effective manner.
On a more positive note, generative AI has a myriad of promising potential applications, with the responsibility for its use falling to the companies and individuals that use it.
For one, generative AI can enable individuals and businesses to focus on their areas of expertise, accelerating processes by doing the work that isn’t an individual or business’s main concern. For example, if my area of expertise is writing a blog, and I need a cover image for an article, my time is not best utilized by spending hours searching for the right picture to display. Instead, a generative AI tool could possibly create the picture I need in a matter of minutes, allowing me to hone my focus on writing.
Similarly, generative AI can significantly enhance scale and automation in various processes to make workflows more efficient, and help an individual/business do their work in a scalable way to produce a more efficient output. Using the same blog example, if I type at 40 words per minute, but speak at 120 words per minute, using an AI dictation tool that converts my speech into text would allow me to publish articles at a much higher rate. Expertise and efficiency, and increased scale and automation, both result in saving time and resources by reducing non-expertise work and allowing for a faster workflow in general.
To wrap up the potential perils and promises, Folkman offered ways on how to stay ahead with generative AI. It is essential for businesses to find unique and valuable data that competitors are not utilizing in order to drive distinction and avoid non-differentiated content. Plus, investing in talent and expertise that deeply understands AI within your organization is critical, so that it may be utilized safely, effectively, and to the maximum benefit of both individuals and businesses as a whole.
Embracing a culture of innovation is necessary, even though some attempts may fail; therefore, commitment to improving and implementing AI technology is key to staying ahead. Folkman’s final point suggests creating clear objectives: clearly define what you want generative AI technology to achieve for your business, continuously monitor its performance, and be willing to adapt strategies based on evolving goals.
In conclusion, Folkman’s strategies provide helpful insights on best practices that individuals and businesses can employ to maximize its applicability. And while official legislation on generative AI may not move at the same speed of innovation, businesses are still responsible for ensuring that generative AI’s applications are ethical and protect the privacy of customers. This gray area before clear legislation is enacted can still be navigated in a way that mitigates risk and potential perils, for the benefit of businesses and individuals alike. By bridging modern technical capabilities with business acumen and responsible usage, we can work towards safely incorporating generative AI to leverage its value.
At Kubrick, we help organizations to evolve and embrace next-generation technology with the skills that deliver change and impact. Our unique approach to hiring and training talent from a wide range of backgrounds allows us to deploy consultants, equipped with today’s most sought-after skills at the intersection of data, AI, and governance, to solve your business’s data challenges and emergencies. To learn more about how Kubrick can support your team to navigate the changing technology landscape, get in touch: speaktous@kubrickgroup.com
Latest insights
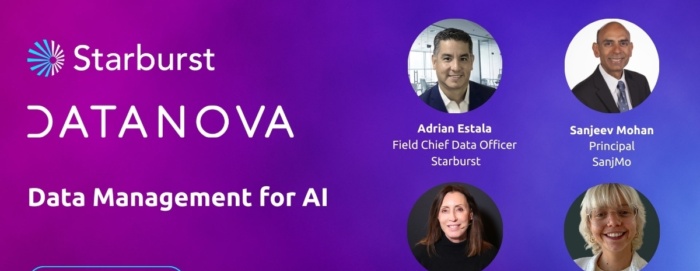